This post is part of a series highlighting the summer projects from our office’s undergraduate research assistants. The sixth part of this series is by Geneva Ely, a senior double majoring in Meteorology and Environmental Sciences with a concentration in Air Quality at NC State.
This summer I started working on a research project to correct biases (overestimates and underestimates) in Multi-Sensor Precipitation Estimates (MPE) within North Carolina. These precipitation estimates are calculated using WSR-88D Doppler Radar derived precipitation values calibrated using National Weather Service (NWS) hourly weather stations.
MPE is so useful because it can give you an estimation of precipitation in a location that is not represented by a rain gauge. This functionality is useful for streamflow management modeling. However, sometimes these estimates miss the mark when compared to surface rain gauges not used in the MPE calculation. My project is to bias correct the MPE data set using daily station observation not used in the original calculation of MPE, such as NWS COOP stations.
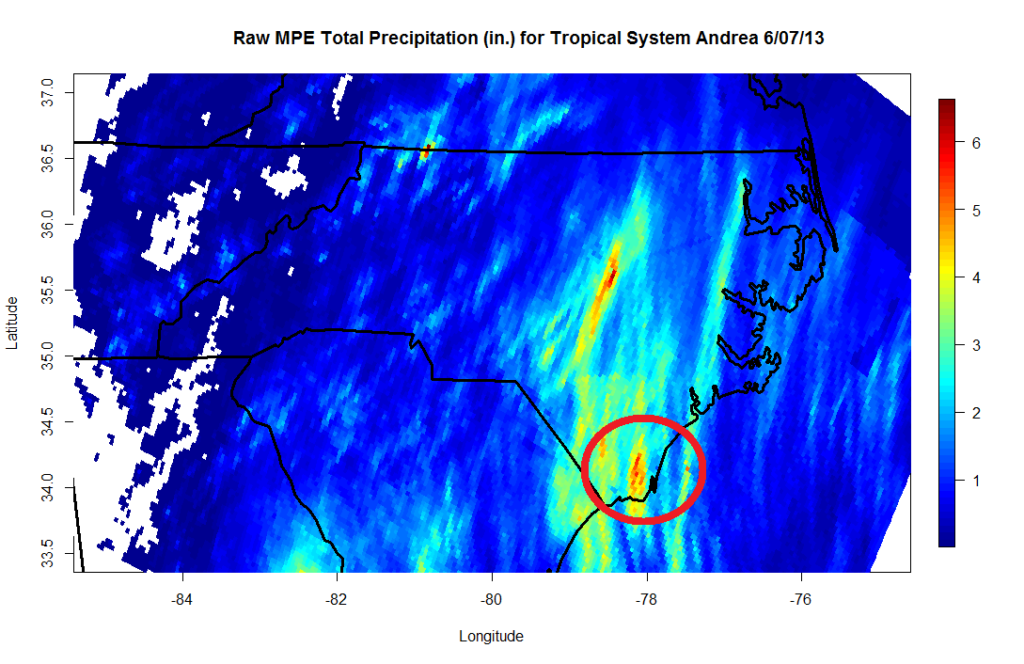
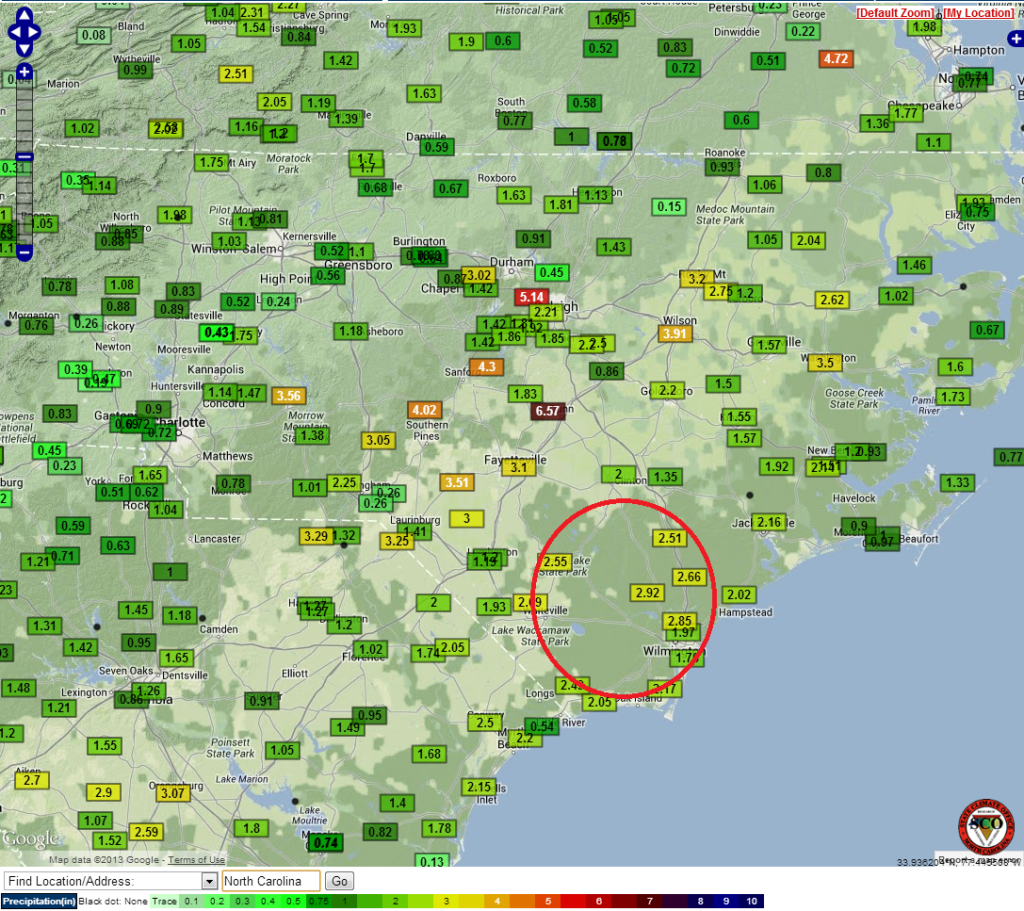
Please note not all of the above stations define a day the same way. For more information, see our previous blog post on this topic!
Right off the bat we see can see the difference in resolution with MPE showing a much finer scale than the point measurements of the COOP map. Next we can see an area of higher values on the MPE map around Brunswick, New Hanover and Pender counties that is absent from the COOP map (location circled in red). MPE indicates that it rained 5 to 6 inches, while ground measurements only show 3 inches of rainfall at most. Who do we trust when there is such a discrepancy?
Normally we trust the ground measurements, which means the MPE require a little tweaking. That is where I come in! I am working to apply a Bayesian spatial technique (Bayesian Spatial Quantile Regression, for those of you with a background in statistics) to attempt correcting the differences between the COOP station data and the MPE data. That way we can have a better quality, high-resolution data set to use in the products we offer.